Alvaria Blog
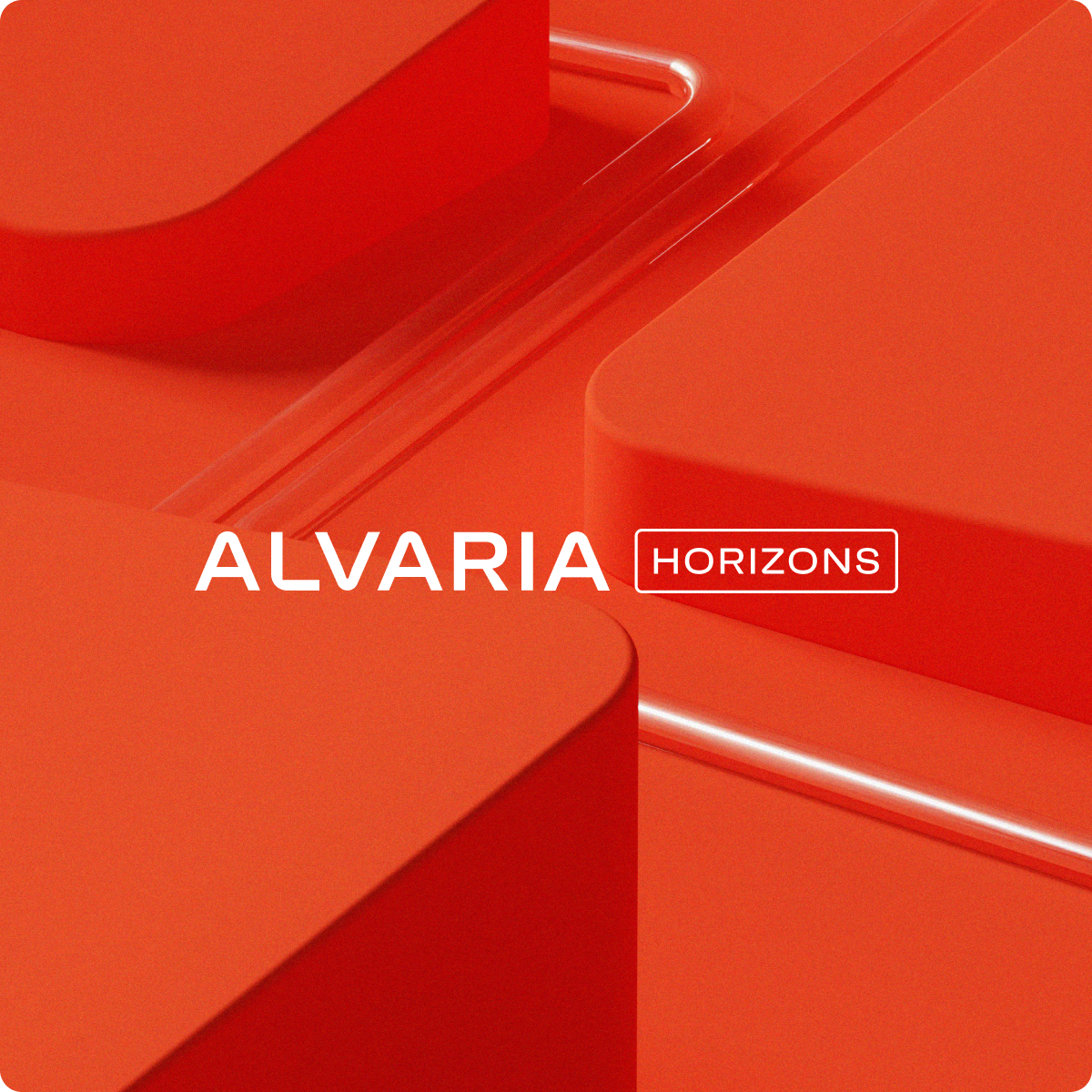
Introducing Alvaria Horizons, our innovation lab dedicated to building a brighter future of work
We’re thrilled to announce Alvaria Horizons, a lab dedicated to pioneering new ideas and building innovative technologies. We launched this space to bring new ideas and products to the continuously shifting workplace landscape, taking an even deeper dive into new ways to build a brighter future of work. Our team is energized by the idea of blending Alvaria’s innovative legacy with renewed curiosity and openness, bringing forward new tools and perspectives that elevate the way humans and technology collaborate.
Introducing Alvaria Horizons, our innovation lab dedicated to building a brighter future of work
Thank you! Your submission has been received!
Oops! Something went wrong while submitting the form.
Case studies

February 16, 2024
Alvaria and CallMiner Redefine Innovative Partnership Dynamics
Alvaria and CallMiner Redefine Innovative Partnership Dynamics
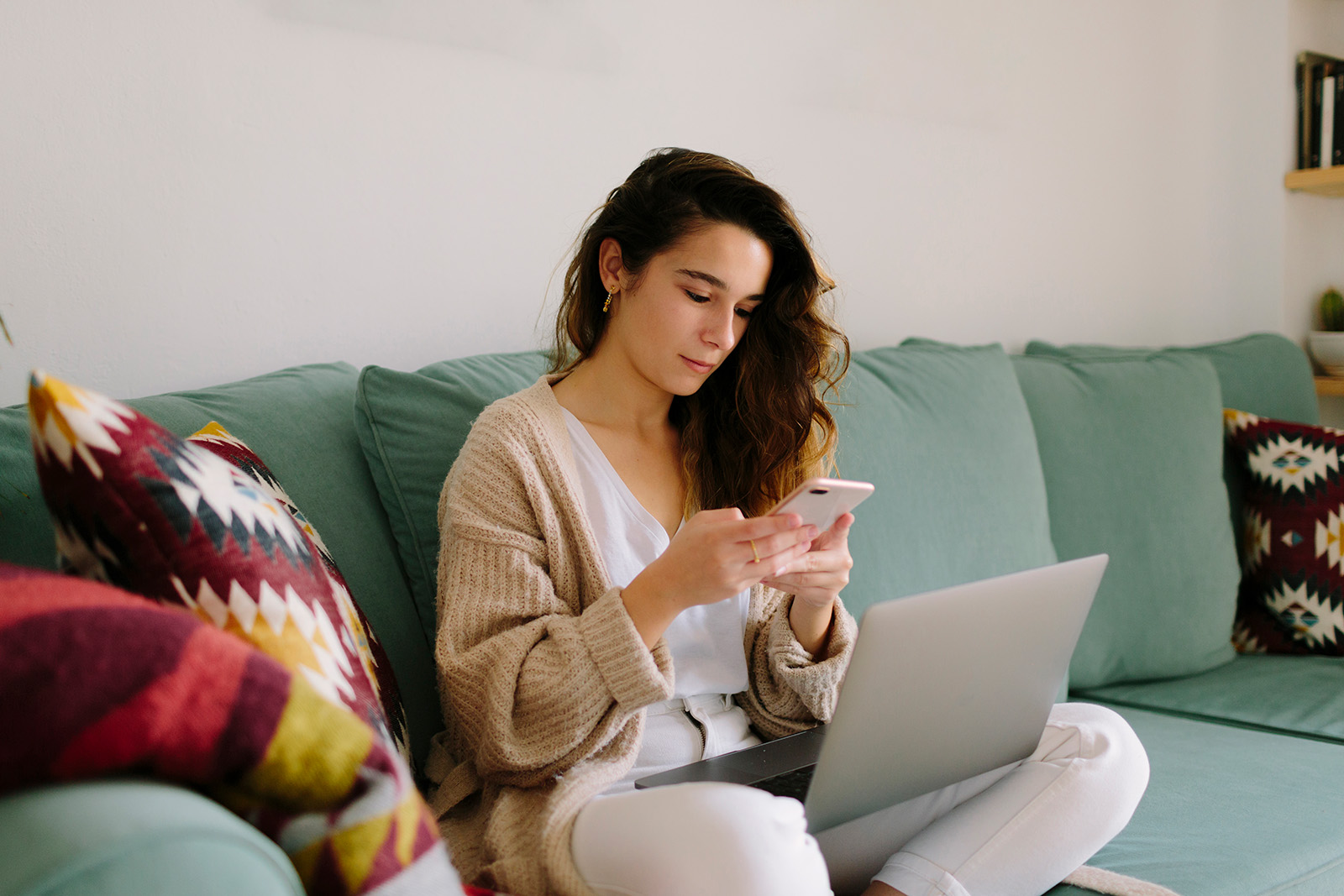
December 19, 2023
Alvaria Workforce Engagement Version 23: Bringing Powerful Features for Enhanced Efficiency and Engagement
Alvaria Workforce Engagement Version 23: Bringing Powerful Features for Enhanced Efficiency and Engagement

October 17, 2023
Navigating the Contact Center Jungle: Your Guide to Contact Center Infrastructure Adventures
Navigating the Contact Center Jungle: Your Guide to Contact Center Infrastructure Adventures
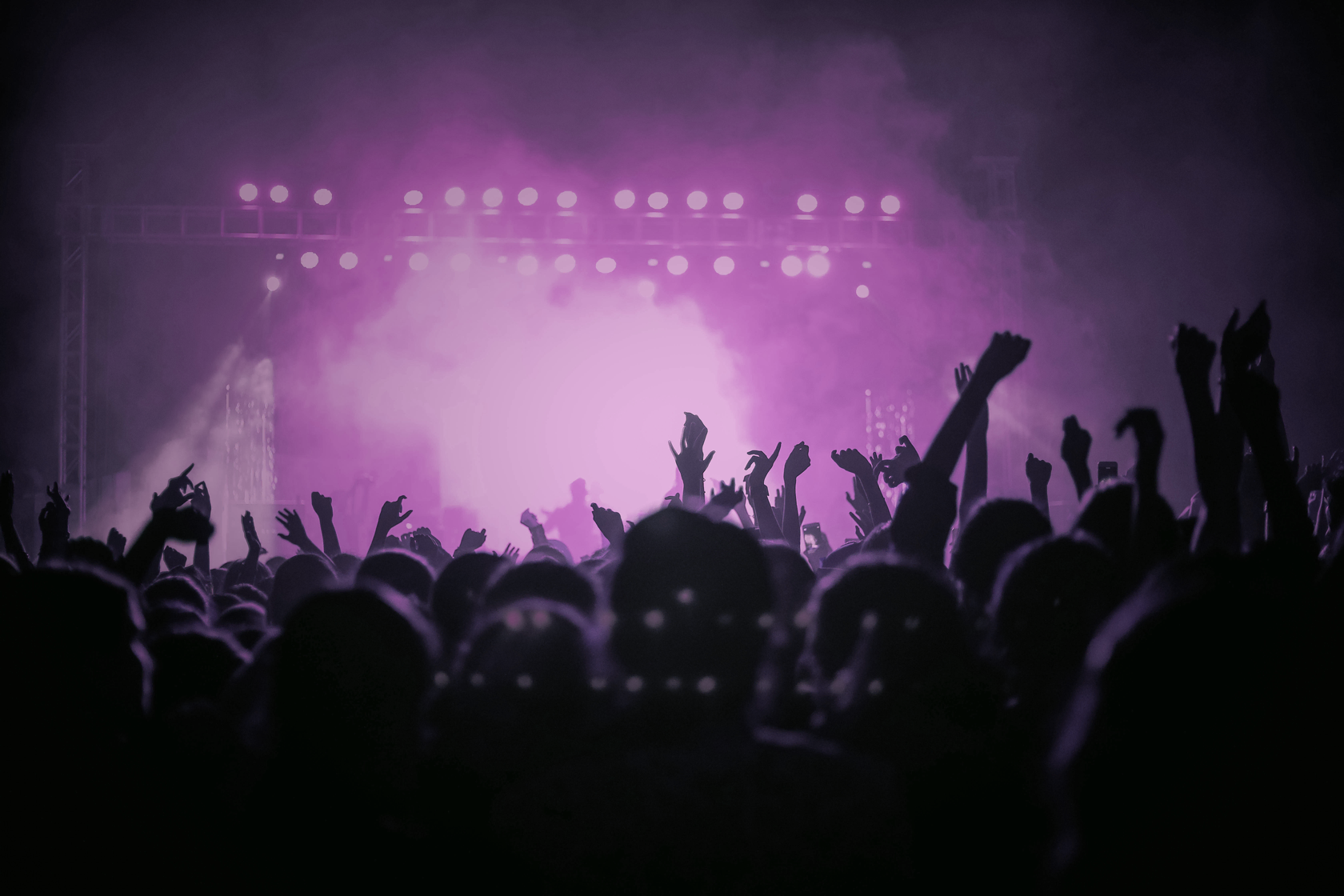
October 10, 2023
What Can We Learn from Taylor Swift About Customer Experience?
What Can We Learn from Taylor Swift About Customer Experience?
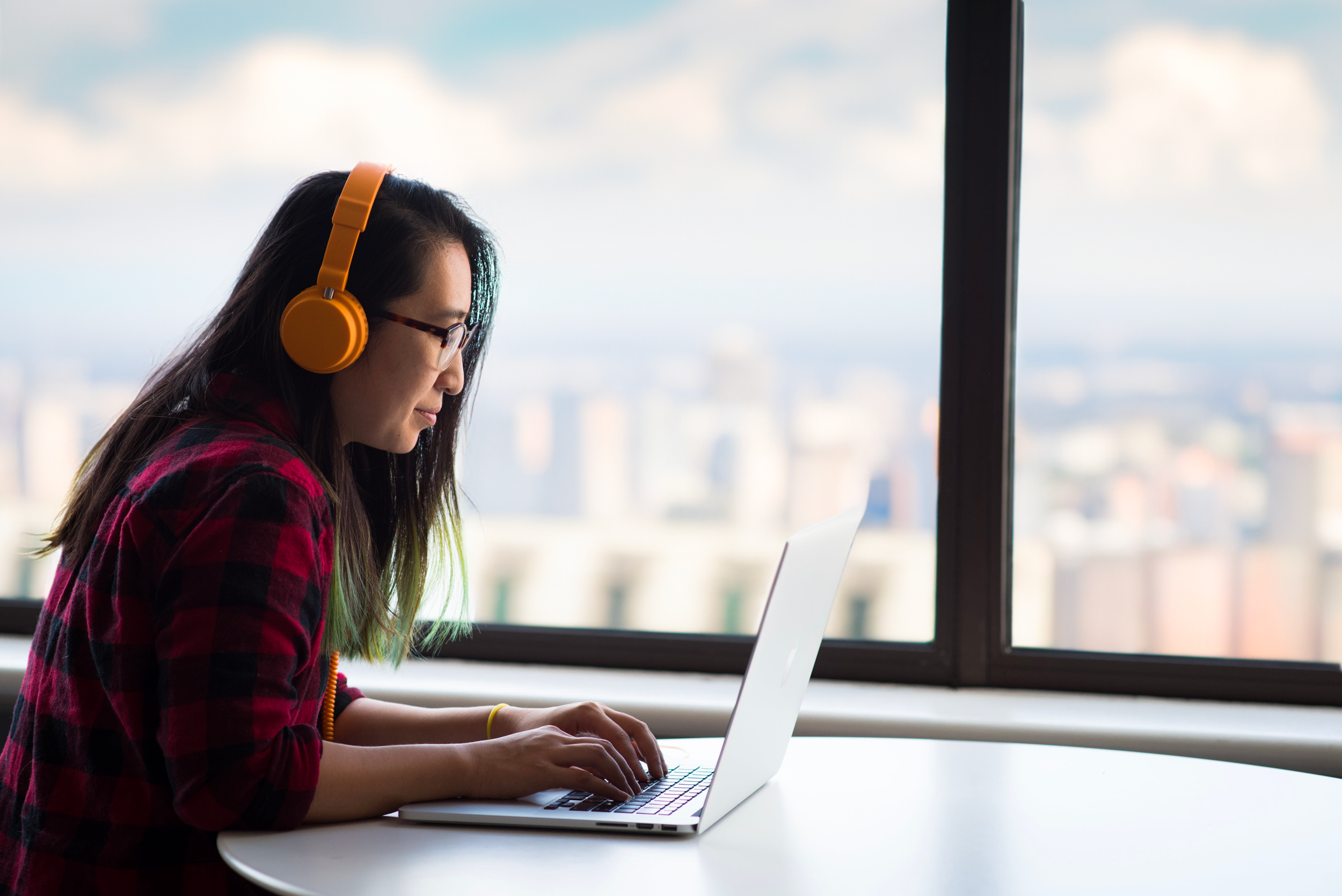
September 27, 2023
The Journey to Workforce Engagement Management in the Cloud
The Journey to Workforce Engagement Management in the Cloud
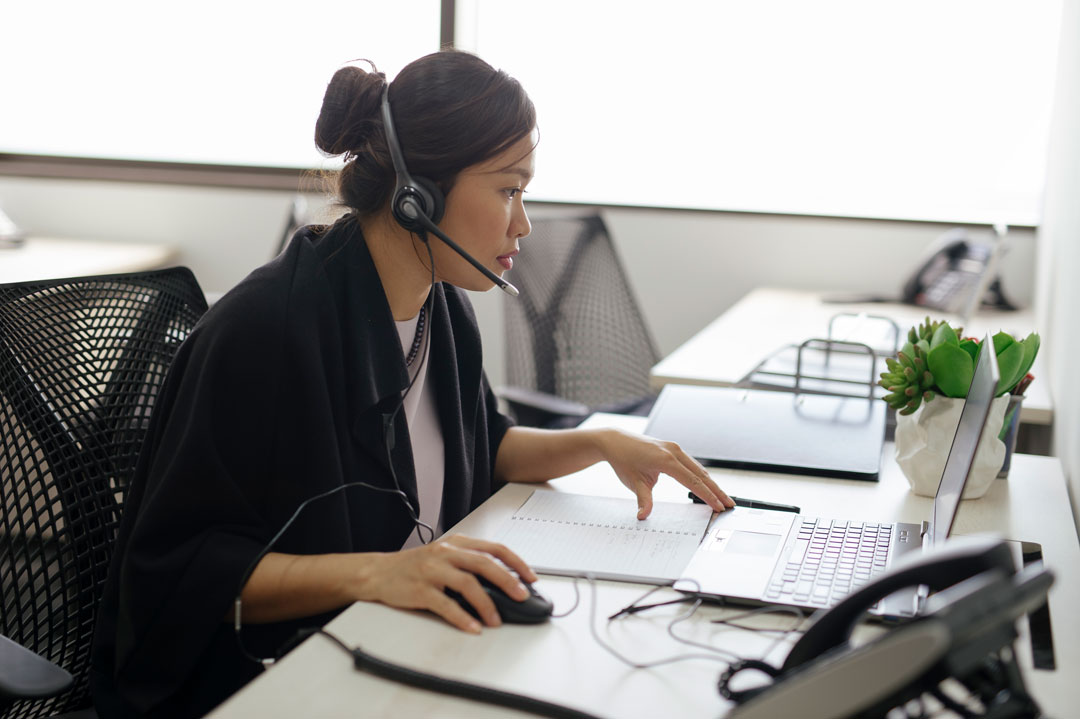
September 26, 2023
Rethinking Roles – Agents in the Age of Automation
Rethinking Roles – Agents in the Age of Automation
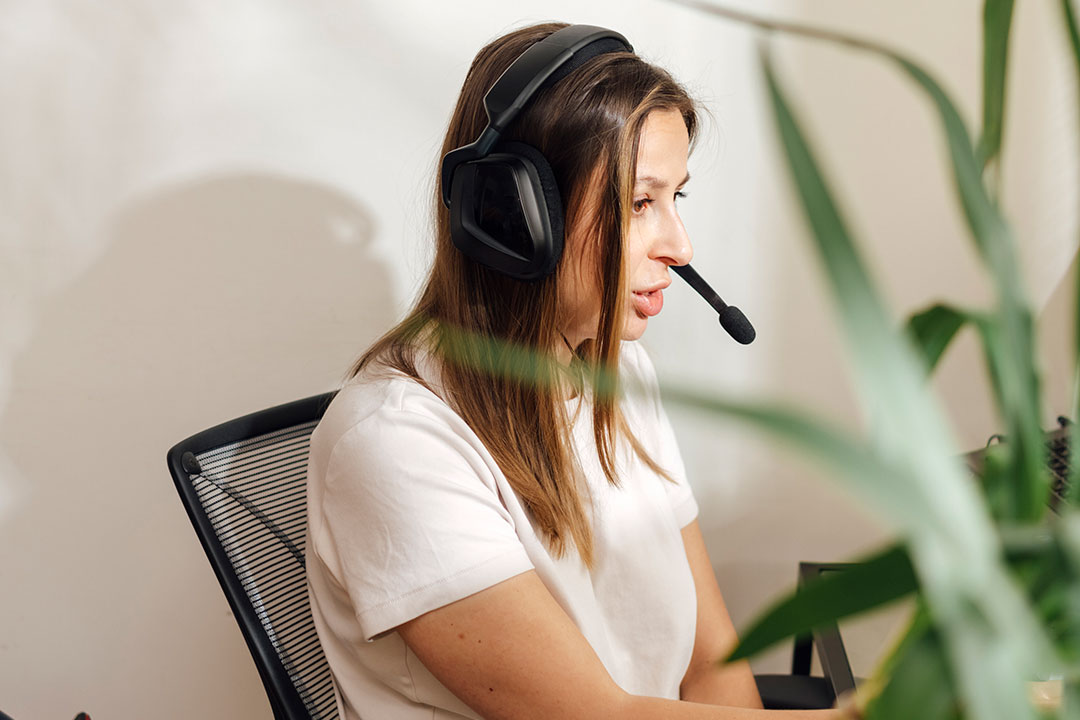
September 19, 2023
Keeping it Real: Agent Excellence in the Age of AI
Keeping it Real: Agent Excellence in the Age of AI

August 22, 2023
The New Characteristics of Workforce Engagement
The New Characteristics of Workforce Engagement
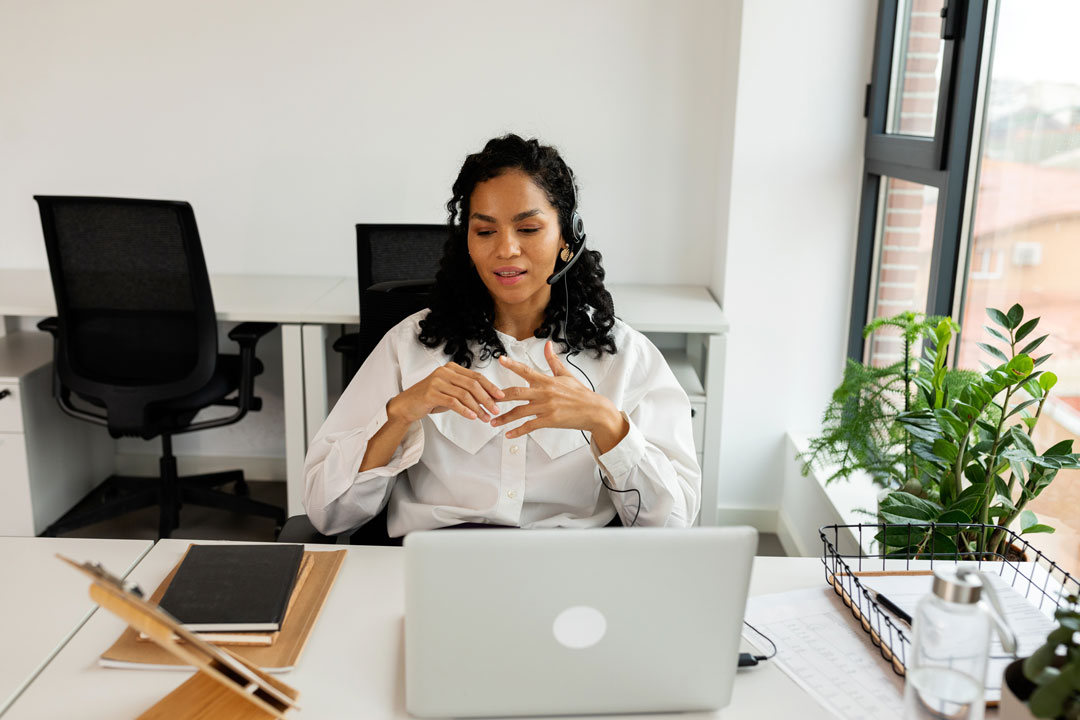
July 19, 2023
Preparing your Agents for New Outbound Challenges by Understanding EX
Preparing your Agents for New Outbound Challenges by Understanding EX
See what all the buzz is about Alvaria in the News
Visit News Page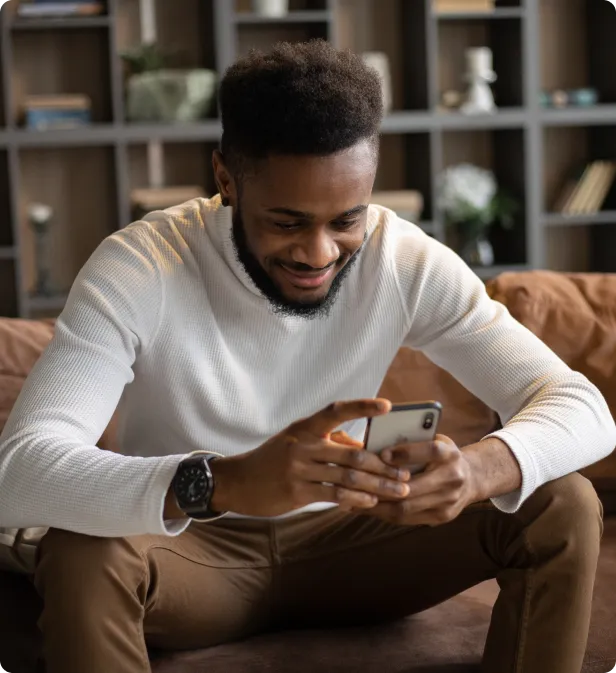